We taught the world the value of big data with open source, and our strong beliefs in the value of open source, open standards, and open markets are driving the next wave of innovation.
Open source innovation
Some vendors consume the open source community’s activity; others help drive it. Cloudera leads the data, analytics, and AI platform evolution by creating, contributing, and supporting new and differentiated capabilities that meet your requirements for security, scale, and usability.
Curation of open standards
Cloudera has a long and proven track record of identifying, curating, and supporting open standards (including Apache Iceberg, Apache Nifi, and Apache Ozone) that provide the mainstream, long-term architecture upon which both new and existing enterprise use cases are built.
Highest enterprise demands
To ensure the best customer experience, Cloudera invests significant resources in multi-dimensional testing on real workloads before releases, implements and maintains security policies based on industry best practices and regulatory requirements, and supports the platform through extensive involvement in the open source community.
Cloudera Data Flow
powered by Apache Nifi
Cloudera Data Flow is a cloud-native data service powered by Apache NiFi that facilitates universal data distribution by streamlining the end-to-end process of data movement.
Cloudera Object Store
powered by Apache Ozone
In the data center, Cloudera Object Store delivers high density and cloud-native object storage, for data storage at tremendous scale and efficiency with Apache Ozone.
Cloudera Open Data Lakehouse
powered by Apache Iceberg
Cloudera’s data lakehouse is built on Iceberg, the industry-standard open table format, delivering high performance at any scale and integration with the widest ecosystem of compute engines.
Cloudera is committed to the open source ethos, including the success of open source projects and open source communities.
200+
Apache committer seats
50+
PMC seats
>55
Projects involved
Our open source ecosystem
The Cloudera platform leverages a large ecosystem of open source projects and technologies that come together to create a true hybrid platform for data, analytics, and AI. Cloudera has an extensive and proven track record in creating, contributing, and supporting open source innovation for enterprise implementation.
Apache Accumulo
A sorted, distributed key-value store with cell-based access control.
Apache Airflow
Workflow management platform for data engineering pipelines.
Apache Arrow
Software framework for developing columnar data processing analytics.
Apache Atlas
Agile enterprise regulatory compliance through metadata.
Apache Avro
Row-oriented remote procedure call and data serialization framework.
Apache Calcite
Framework for building databases and data management systems.
Apache Flink
A real-time stream processing framework for big data analytics and applications.
Apache Hadoop
A distributed storage and processing framework for large-scale data processing tasks.
Apache HBase
A non-relational (NoSQL) database that runs on top of HDFS.
Apache Hive
The de facto standard for SQL queries in Hadoop.
Apache Iceberg
An open table format for large-scale analytics, delivering the reliability and simplicity of SQL tables.
Apache Impala
The open source, analytic MPP database for Apache Hadoop that provides the fastest time-to-insight.
Apache Kafka
A fast, scalable, fault-tolerant messaging system
Apache Knox Gateway
A secure entry point for Hadoop clusters.
Apache Kudu
Storage for use cases that require fast analytics on rapidly changing data.
Apache Livy
REST interface for Spark clusters.
Apache NiFi
A real-time integrated data logistics and simple event processing platform.
Apache Oozie
The blueprint for enterprise Hadoop, including its original data storage and data processing layers.
Apache Orc
Column-oriented data storage format optimized for read operation.
Apache Ozone
Highly scalable distributed object store with S3 compatible APIs.
Apache Parquet
Column-oriented data storage format optimized for WORM operation.
Apache Phoenix
A massively parallel relational database engine supporting OLTP for Hadoop using Apache HBase.
Apache Ranger
Comprehensive security for Enterprise Hadoop.
Apache Solr
Rapid indexing & search on Hadoop.
Apache Spark
Spark adds in-Memory Compute for ETL, AI, and data science workloads to Hadoop.
Apache Sqoop
Efficiently transfers bulk data between Apache Hadoop and structured datastores.
Apache Tez
A Framework for YARN-based, Data Processing Applications In Hadoop.
Apache YARN
The Architectural Center of Enterprise Hadoop.
Apache Zeppelin
A completely open web-based notebook that enables interactive data analytics.
Apache ZooKeeper
An open source server that reliably coordinates distributed processes.
Docker
Containerization through OS-level virtualization.
Hue
An open source SQL Workbench for Data Warehouses.
Tensorflow
Software library for machine learning and artificial intelligence.
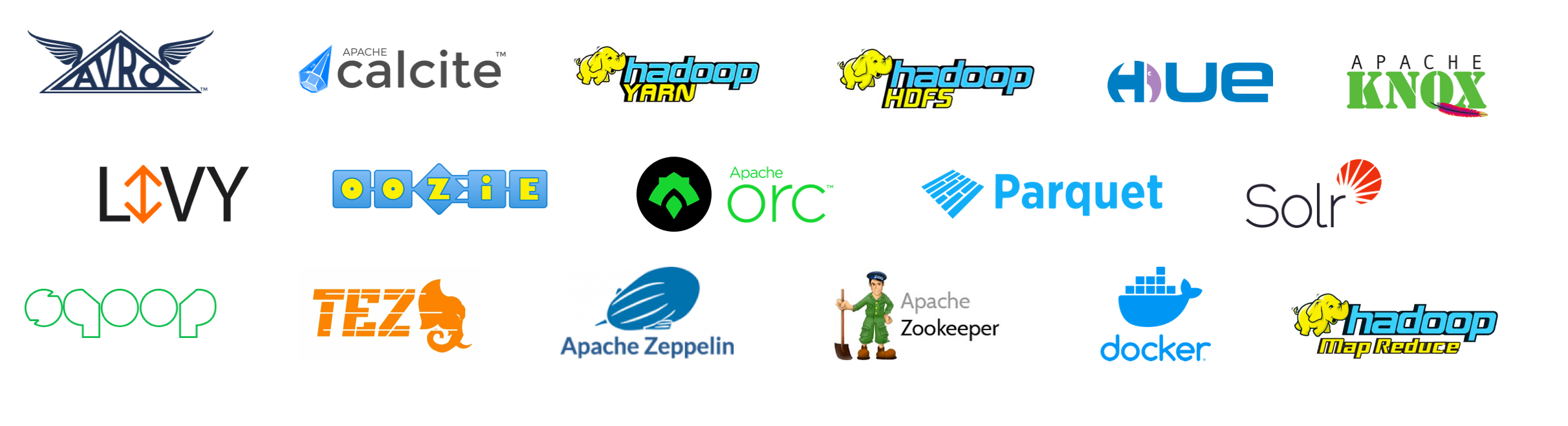