Recently added
2025 trends for data and AI
Hear three data and AI leaders discuss trends and their perspectives on the business impact.
The state of enterprise AI and modern data architectures
New comprehensive report based on a global survey of 600 IT leaders
Data Architecture Series: The open data lakehouse
Building a modern data lakehouse with Cloudera
Popular resources
Recent blogs
Recent success stories
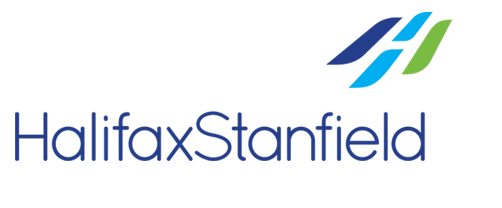
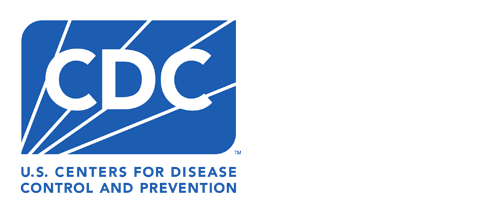
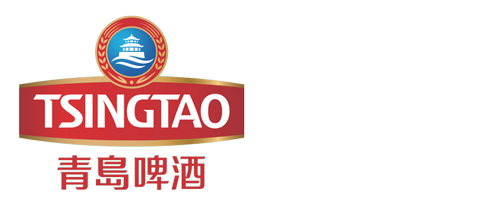