Hear how leading companies thrive with Cloudera
Celebrating 10+ Years of Data Impact by Cloudera Customers!
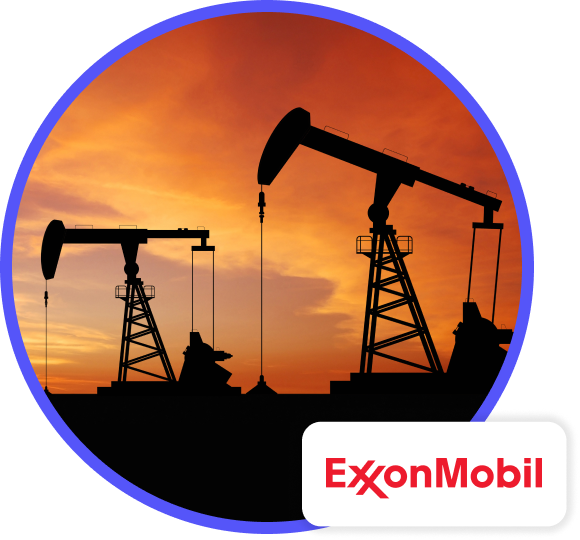
Alicia Morrison, Director of Data Science, Mercy Corps
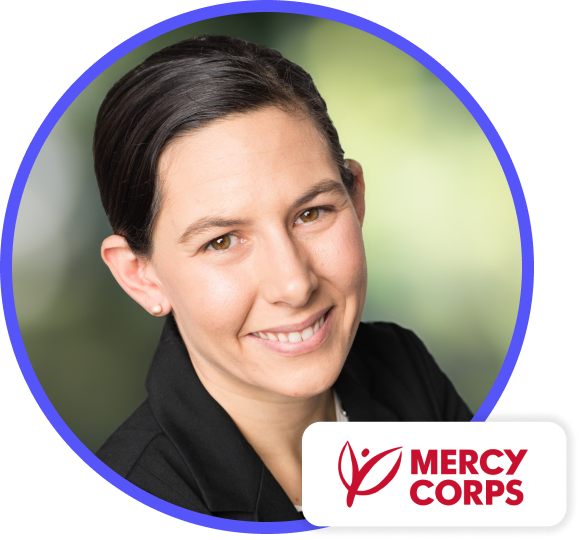
Delio Amato, Group Chief Architect Officer, Geodis
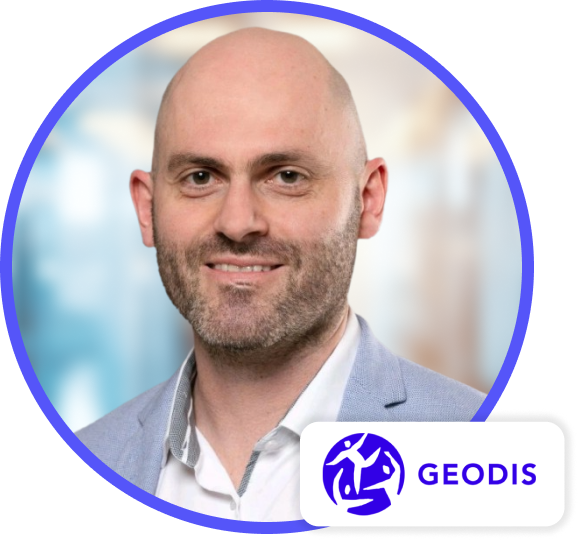
Donald MacDonald, Head of Group Data Office, OCBC Bank
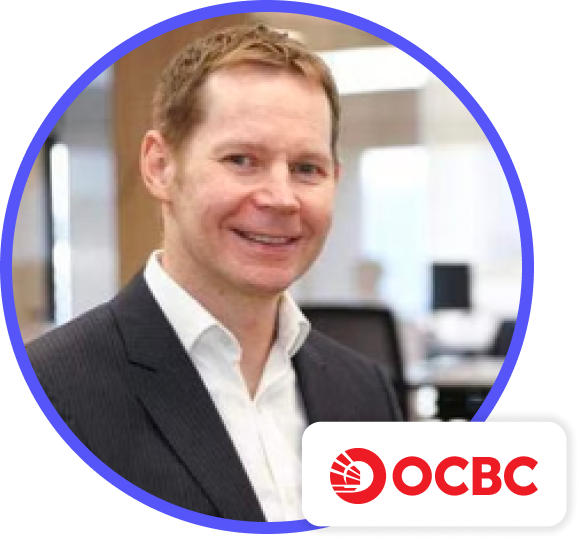
Laura Koormann, Application Manager ContiConnect & IoT, Continental Tires
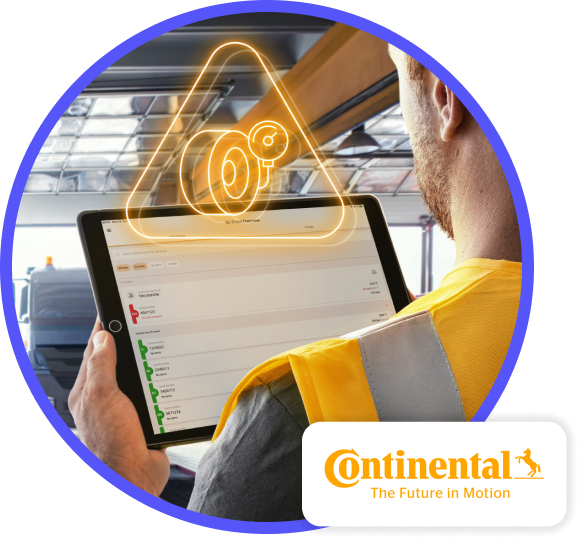
Featured customers
Continental
Transforming fleet services with streaming analytics and Cloudera on public cloud
BT Group
Enhancing customer experience and reducing costs with a data lakehouse on Cloudera
SAIC-Volkswagen
Driving innovation at scale for vehicle data monitoring and reducing costs with Cloudera
Center of Disease Control
Advancing Public Health with Advanced Data Analytics with Better Disease Tracking
Amdocs
Monetizing telco services at scale with real-time billing data on premises and on cloud
FairConnect
Revolutionizing the industry with IoT data for "connected" solutions and Cloudera on AWS
See how Cloudera enables organizations to delight customers around the world every day.
Be the next
success story
Elevate your profile, connect with data leaders, and promote your team's accomplishments with data, analytics, and AI.
Industry leaders at events and in the news
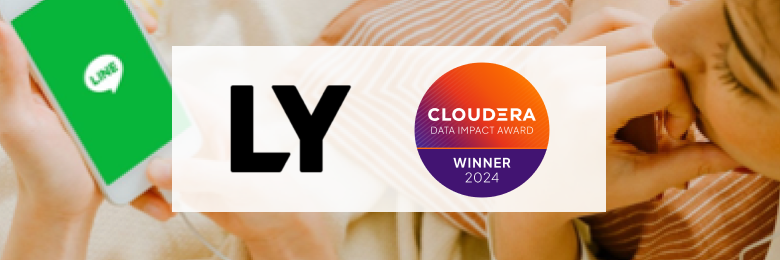